5 Ways To Low Rank
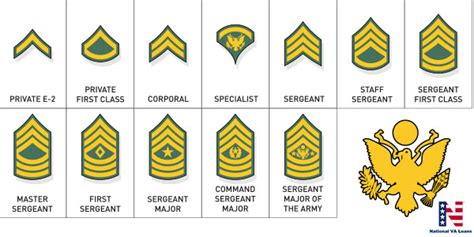
Introduction to Low-Rank Approximations
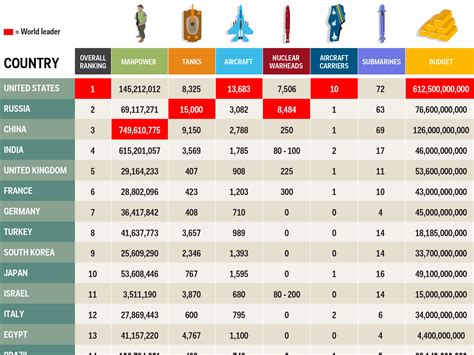
Low-rank approximations are a fundamental tool in data analysis and machine learning, allowing for the reduction of dimensionality in large datasets. This technique is essential for improving computational efficiency and reducing noise in the data. In this blog post, we will explore five ways to achieve low-rank approximations, highlighting their applications, benefits, and implementation details.
1. Singular Value Decomposition (SVD)
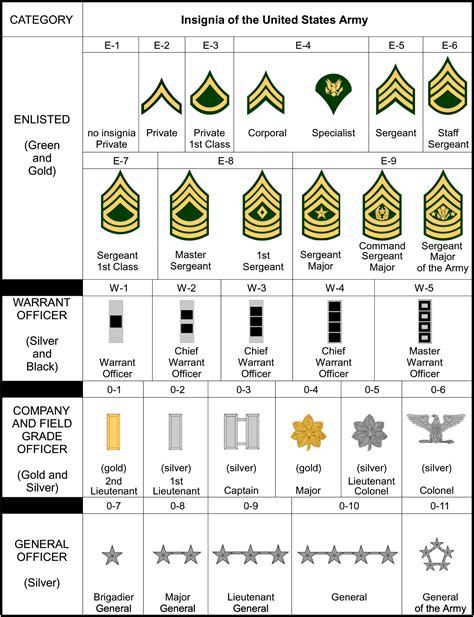
Singular Value Decomposition (SVD) is a widely used technique for low-rank approximation. It factorizes a matrix into the product of three matrices: U, Σ, and V. By retaining only the top k singular values and the corresponding singular vectors, we can obtain a low-rank approximation of the original matrix. SVD is particularly useful for image compression and latent semantic analysis.
2. Non-negative Matrix Factorization (NMF)

Non-negative Matrix Factorization (NMF) is another popular technique for low-rank approximation. It factorizes a non-negative matrix into the product of two non-negative matrices: W and H. NMF is commonly used in text analysis and recommendation systems. By imposing non-negativity constraints, NMF can produce more interpretable and meaningful results.
3. Randomized SVD (RSVD)
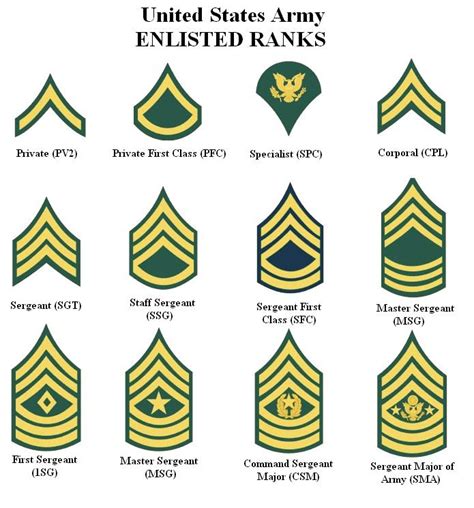
Randomized SVD (RSVD) is a faster and more efficient alternative to traditional SVD. It uses random sampling to approximate the singular values and singular vectors of a matrix. RSVD is particularly useful for large-scale datasets where traditional SVD is computationally expensive. By reducing the computational cost, RSVD enables low-rank approximations to be applied to a wider range of applications.
4. CUR Decomposition
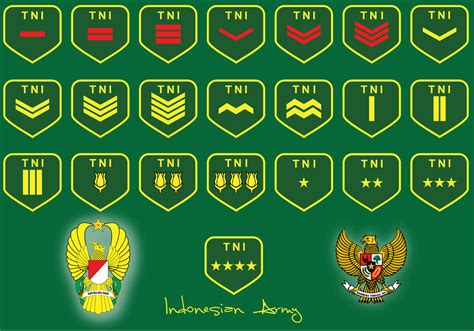
CUR decomposition is a low-rank approximation technique that factorizes a matrix into the product of three matrices: C, U, and R. It is commonly used in data mining and information retrieval. CUR decomposition can produce more accurate results than SVD, especially when the data contains outliers or noise.
5. Low-Rank Approximation via Sparse Coding

Low-rank approximation via sparse coding is a technique that uses sparse coding to approximate a matrix. It represents each data point as a linear combination of a few basis elements. This technique is particularly useful for image denoising and feature extraction. By imposing sparsity constraints, sparse coding can produce more robust and generalizable results.
🔍 Note: The choice of low-rank approximation technique depends on the specific application and the characteristics of the data.
The following table summarizes the five low-rank approximation techniques discussed in this blog post:
Technique | Description | Applications |
---|---|---|
SVD | Factorizes a matrix into U, Σ, and V | Image compression, latent semantic analysis |
NMF | Factorizes a non-negative matrix into W and H | Text analysis, recommendation systems |
RSVD | Approximates SVD using random sampling | Large-scale datasets, efficient computation |
CUR decomposition | Factorizes a matrix into C, U, and R | Data mining, information retrieval |
Low-rank approximation via sparse coding | Represents each data point as a linear combination of basis elements | Image denoising, feature extraction |
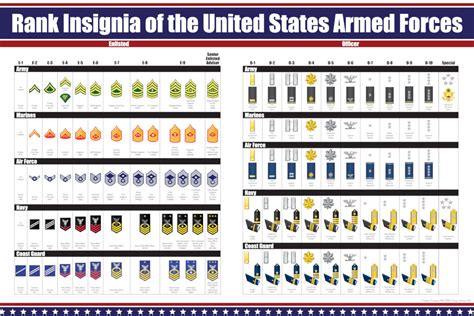
In summary, low-rank approximations are a powerful tool for reducing dimensionality and improving computational efficiency in data analysis and machine learning. The five techniques discussed in this blog post - SVD, NMF, RSVD, CUR decomposition, and low-rank approximation via sparse coding - each have their own strengths and weaknesses, and the choice of technique depends on the specific application and characteristics of the data. By understanding these techniques and their applications, practitioners can unlock the full potential of low-rank approximations and drive innovation in their respective fields.
What is low-rank approximation?
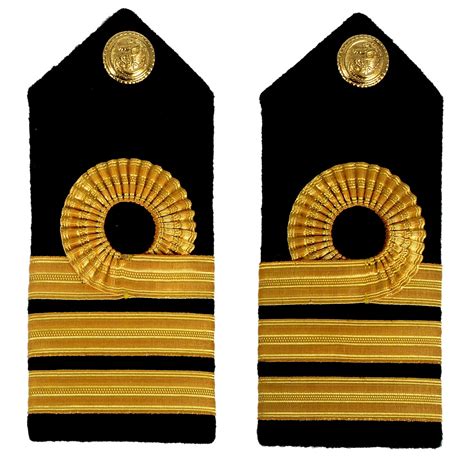
+
Low-rank approximation is a technique used to reduce the dimensionality of a matrix by approximating it with a lower-rank matrix.
What are the benefits of low-rank approximation?
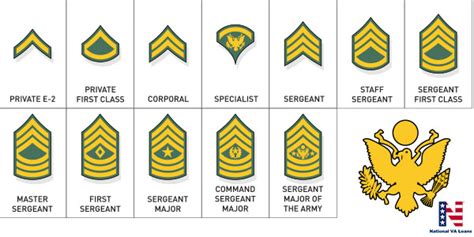
+
The benefits of low-rank approximation include improved computational efficiency, reduced noise, and improved interpretability of the data.
What are some common applications of low-rank approximation?
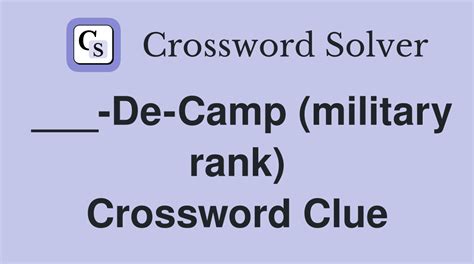
+
Common applications of low-rank approximation include image compression, latent semantic analysis, text analysis, recommendation systems, and feature extraction.
Related Terms:
- Military rank in the world
- Military rank list
- Highest military rank
- Military rank 1960
- Military rank Indonesia
- U S military rank